In today’s data-driven world, Business Intelligence (BI) and Data Analytics play pivotal roles in helping organizations derive actionable insights from vast amounts of data. While often used interchangeably, these terms represent distinct disciplines with unique objectives and methodologies. This article aims to unravel the complexities surrounding Business Intelligence and Data Analytics, exploring their differences, applications, benefits, and future trends.
KEY HIGHLIGHTS
- Business Intelligence enables organizations to monitor key performance indicators (KPIs), track business trends, and identify opportunities or risks in real-time
- Data Analytics enables organizations to gain a competitive edge by leveraging data-driven insights
- Business Intelligence typically relies on structured data from internal sources such as transactional databases and enterprise systems
- Data Analytics encompasses a broader range of data sources, including structured, semi-structured, and unstructured data from internal and external sources
What is Business Intelligence (BI)?
2.1 Definition and Overview:
Business Intelligence (BI) involves the process of collecting, analyzing, and interpreting data to support business decision-making. It encompasses technologies, applications, and practices for turning raw data into meaningful insights to drive strategic planning and operational improvements.
2.2 Key Components:
BI solutions typically comprise data warehousing, data integration, data visualization, and reporting tools. These components work together to facilitate data-driven decision-making at various levels of an organization.
2.3 Importance in Decision Making:
BI enables organizations to monitor key performance indicators (KPIs), track business trends, and identify opportunities or risks in real-time. By providing access to accurate and timely information, BI empowers stakeholders to make informed decisions that drive business growth and competitive advantage.
Understanding Data Analytics
3.1 Definition and Scope:
Data Analytics involves the exploration, interpretation, and communication of meaningful patterns and insights derived from data. It encompasses a wide range of techniques, including statistical analysis, predictive modeling, and machine learning, to extract actionable insights from structured and unstructured data sources.
3.2 Techniques and Processes:
Data Analytics involves various processes such as data cleansing, data modeling, exploratory data analysis, and predictive modeling. These techniques aim to uncover hidden patterns, trends, and correlations within the data to support decision-making and strategic planning.
3.3 Applications in Various Industries:
Data Analytics finds applications across diverse industries, including finance, healthcare, retail, and manufacturing. From customer segmentation and market analysis to risk management and predictive maintenance, Data Analytics enables organizations to gain a competitive edge by leveraging data-driven insights.
Business Intelligence vs Data Analytics: A Comparative Analysis
4.1 Objectives and Focus:
While both BI and Data Analytics aim to leverage data for informed decision-making, BI primarily focuses on historical and current data to monitor performance and support operational activities. In contrast, Data Analytics emphasizes predictive and prescriptive analytics to forecast future trends and drive strategic initiatives.
4.2 Data Sources and Processing:
BI typically relies on structured data from internal sources such as transactional databases and enterprise systems. Data Analytics, on the other hand, encompasses a broader range of data sources, including structured, semi-structured, and unstructured data from internal and external sources.
4.3 Tools and Technologies:
BI tools are designed for easy access to predefined reports and dashboards, enabling users to monitor KPIs and track performance metrics. Data Analytics tools, on the other hand, offer advanced analytics capabilities, including statistical analysis, data mining, and machine learning algorithms, to uncover deeper insights from the data.
4.4 Decision-making Support:
BI provides a retrospective view of business performance, helping stakeholders understand what happened and why. In contrast, Data Analytics offers a forward-looking perspective, enabling organizations to predict future outcomes and make proactive decisions to capitalize on opportunities or mitigate risks.
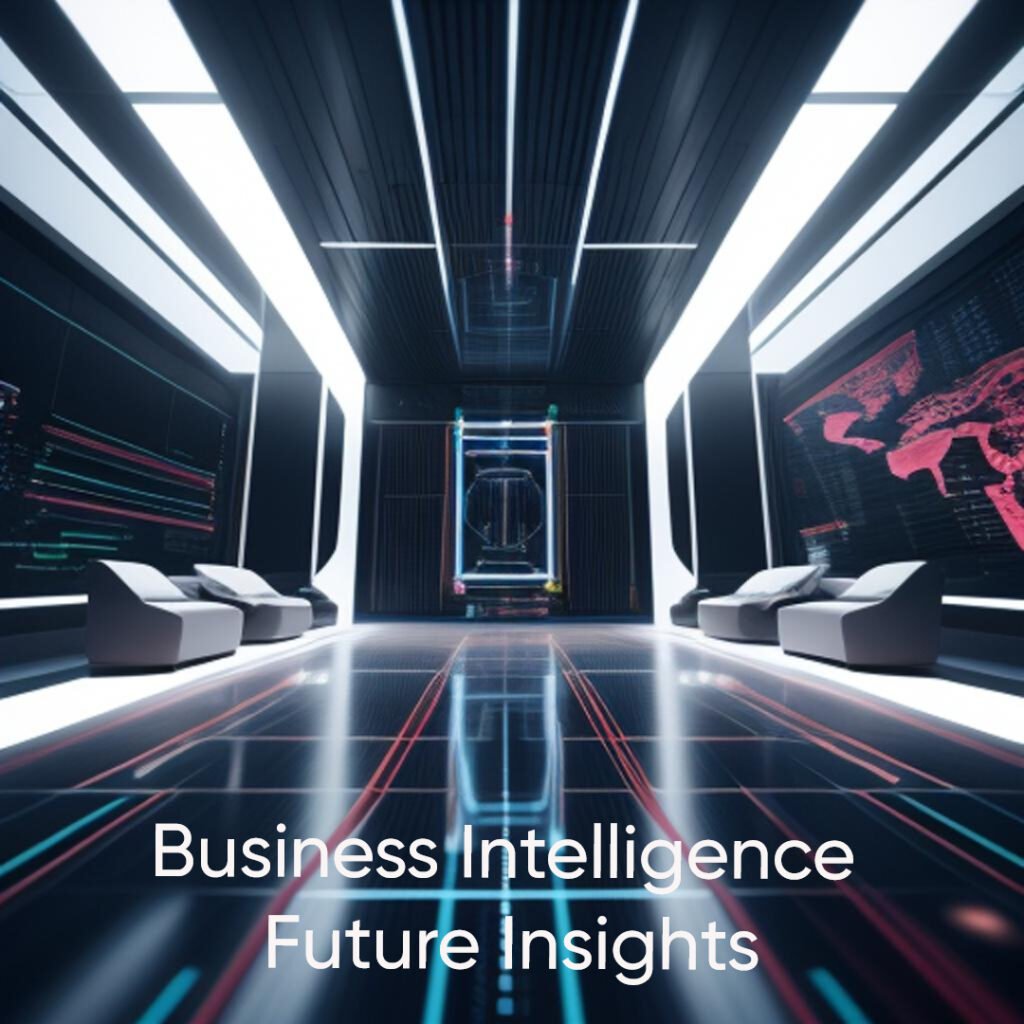
How to Implement Business Intelligence and Data Analytics
5.1 Establishing Goals and Objectives:
Define clear objectives and key performance indicators (KPIs) that align with the organization’s strategic priorities and business objectives.
5.2 Data Collection and Integration:
Ensure data quality and integrity by implementing robust data collection and integration processes, incorporating data from disparate sources into a centralized data repository.
5.3 Analysis and Visualization:
Utilize advanced analytics techniques and visualization tools to analyze data, identify patterns, trends, and outliers, and communicate insights effectively to stakeholders.
5.4 Actionable Insights and Reporting:
Translate insights into actionable recommendations and strategic initiatives, delivering reports and dashboards that provide stakeholders with timely and relevant information to support decision-making.
Benefits of Business Intelligence and Data Analytics
6.1 Improved Decision Making:
BI and Data Analytics enable organizations to make data-driven decisions based on accurate, timely, and relevant information, leading to improved operational efficiency and strategic outcomes.
6.2 Enhanced Operational Efficiency:
By streamlining processes, optimizing resources, and identifying areas for improvement, BI and Data Analytics drive operational excellence and cost savings across the organization.
6.3 Competitive Advantage:
By leveraging data-driven insights to anticipate market trends, understand customer preferences, and identify growth opportunities, organizations gain a competitive edge in the marketplace.
Challenges and Considerations
7.1 Data Quality and Governance:
Ensuring data quality, integrity, and governance is essential to the success of BI and Data Analytics initiatives, requiring robust data management processes and policies.
7.2 Integration Complexity:
Integrating data from disparate sources and systems can be complex and time-consuming, requiring careful planning and coordination across departments and stakeholders.
7.3 Skills Gap and Training:
Addressing the skills gap and providing ongoing training and development for employees is crucial to building a data-driven culture and maximizing the value of BI and Data Analytics investments.
Future Trends in BI and Data Analytics
8.1 AI and Machine Learning Integration:
The integration of artificial intelligence (AI) and machine learning (ML) technologies into BI and Data Analytics platforms will enable more advanced predictive and prescriptive analytics capabilities.
8.2 Advanced Data Visualization Techniques:
Advancements in data visualization techniques, including augmented reality (AR) and virtual reality (VR), will enable more immersive and interactive data exploration experiences.
8.3 Focus on Real-time Analytics:
The shift towards real-time analytics will enable organizations to gain insights faster, respond to market changes more quickly, and make proactive decisions in dynamic business environments.
Frequently Asked Questions
While Business Intelligence focuses on monitoring and reporting historical and current data to support operational activities, Data Analytics emphasizes predictive and prescriptive analytics to forecast future trends and drive strategic initiatives.
By leveraging data-driven insights, businesses can improve decision-making, enhance operational efficiency, and gain a competitive advantage in the marketplace.
Common challenges include ensuring data quality and governance, addressing integration complexity, and bridging the skills gap through ongoing training and development initiatives.
Future trends include the integration of AI and machine learning technologies, advancements in data visualization techniques, and a focus on real-time analytics to enable faster decision-making and response to market changes.